Presented by:
Editor's note: This article appears in the new E&P newsletter. Subscribe to the E&P newsletter here.
The decision-making process of positioning a new well must include a comprehensive analysis of the target rock properties to be drilled. This analysis is based on the knowledge acquired from vintage wells and any previous studies done for earlier operators in the area or nearby areas. Some concepts like brittleness, porosity and organic matter may be brought to the table during the investigation, and the technical team should not forget about the relation between these elements and the geological context, lithology and stress field.
An idea or sketch of the current structural and stratigraphic geological setting can be established through a qualitative interpretation of the seismic data (picking faults, horizons and studying the relative changes of the stacked amplitude values or stacked seismic amplitude derived attributes). This qualitative model allows users to reconstruct the local tectonic events and depositional environments at any geological time and into a larger or smaller lateral scale.
Therefore, inferences about the fabric/texture of the rocks, distribution of the facies of interest, sealing structures and more can be made to define potential zones for the accumulation of hydrocarbon. However, economical and uncertainty analysis, critical during the decision-making process of positioning new exploratory or development wells, require quantitative data.
Rock mechanical properties can complement the qualitative model. These properties can be estimated from seismic attributes that are calculated via special processes like amplitude versus offset versus azimuth, horizontal transverse isotropic analysis, amplitude versus offset analysis, and model-based pre-stack seismic inversion, among others. Each of those processes requires a singular type of input data and provides specific types of measured quantities, so it is extremely important to have an idea of the properties that play a fundamental role on the selection of a target to be drilled to collect and process the data accordingly.
Delaware Basin case study
In a case study by Tricon Geophysics, an independent seismic processing, imaging and reservoir services company, a deterministic machine learning process based on a multi-attributes crossplot analysis and a rock property predictor was applied to a dataset from the North West Shelf of the Delaware Basin with the goal of translating prestack seismic inversion attributes to meaningful rock properties in the Wolfcamp interval.
The rock physics crossplot analysis of the available well data allowed users to describe the best reservoir in this area like zones with high total porosity and high volume of dolomite but a low volume of calcite (Figure 1). Both Well A and Well B contain a high volume of dolomite. However, Well A exhibits higher porosity than Well B. Consequently, the combination of rock properties in Well A are the most favorable to work as a reservoir. Therefore, it represents the type of rock that should be targeted for new wells and the objective of this study. The volumetric curves used were calculated using a multi-mineral approach that allows users to match simultaneously multiple modeled data (log, cores, XRD and petrographic data) to conditioned input data by varying the fluid content, the volumetric and the mineral composition of the rock until they get a combination that minimizes the difference between both sets of data.
The first step of the rock property predictor, the training, consists of using upscaled conditioned well log data to evaluate the relationship between every target property to be predicted, total porosity and volume of dolomite, and any pair of elastic attributes that could be derived from a seismic inversion.
In this case, a combination of 66 seismic inversion derived attributes, 4,290 crossplot spaces, were evaluated for each of those target properties. The relationship between each pair of elastic attributes and each target property was calculated through a set of axis rotations of every bi-dimensional crossplot space in a spectrum of angles from 0 to 180 degrees, until users find the angle in which the maximum variance of each target property can be modeled in the form of a vector.
No linearity issues were addressed by applying basic mathematical operations to the input attributes.
Then the predictions, or modeled vectors, from each crossplot space were evaluated against each other. During the second step of the rock property prediction, the one with the highest correlation to the target property was scaled to the same dynamic range of each of the target properties.
Finally, the same set of transforms were applied to the same pair of elastic attributes coming out of the prestack seismic inversion attributes to get tri-dimensional distributions of total porosity and volume of dolomite. The mathematical expression for performing the rotation could be expanded to n-dimensions. However, the prestack seismic inversion primary outputs in this case were limited to only two attributes, P-wave and S-wave impedance.
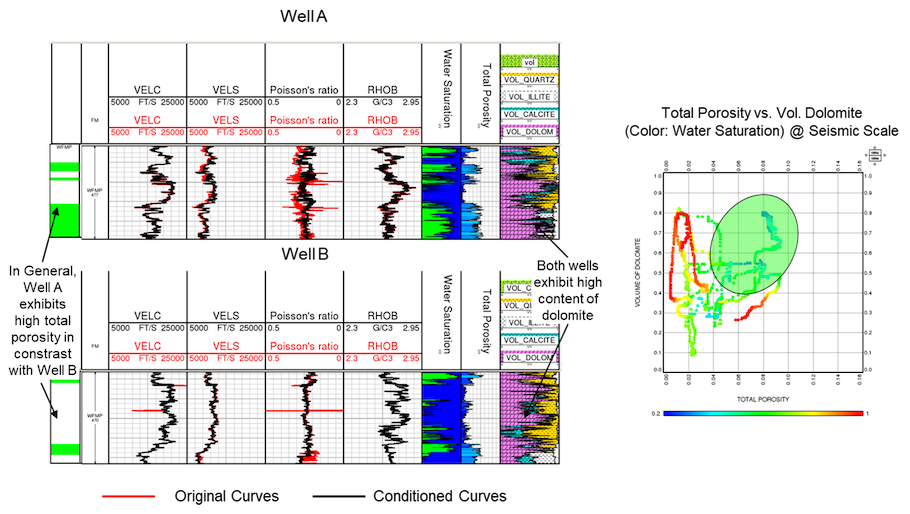
The relationship between the elastic attributes and the rock properties may change laterally and/or vertically between geological formations or inclusively inside the same formation. Consequently, multiple transformations for the same rock property may be needed, and independent rock property cubes need to be merged. In this case, the analysis was constrained in a spatial interval defined by the top of Wolfcamp seismic horizon picked on the seismic amplitude data.
In Figure 2, geo-bodies with the same characteristics to ones observed in the best reservoirs rocks in the available wells were highlighted from the crossplot between the seismic predicted cube of the volume of dolomite and the total porosity in the Wolfcamp interval (pink polygon on the crossplot). These pink geo-bodies were then displayed along with an isochron seismic horizon colored with a light-scape attribute extracted from stacked seismic amplitude data to highlight structural features. In the display, debris flow tracks and fans can be easily identified along the slope and at the base of the shelf as described in multiple geological studies about the area. A sketch of one of these publications is shown on the top right of the figure for reference.
At the bottom right of the same figure, each of the properties were extracted on the Wolfcamp interval and displayed individually from an aerial perspective using a tri-dimensional rendering technique that allows users to set opacity values on each sample as a function of its value. Values of low dolomite and low total porosity were set transparent. The distribution of the high volume of dolomite shows that the debris flow extends to both Well A and Well B. However, the high total porosity only can be seem in the position of Well A as it was observed in the well data. The morphology of the geo-bodies looks geologically correct, and no potential bias for using the same well data as input to the model-based seismic inversion was observed.
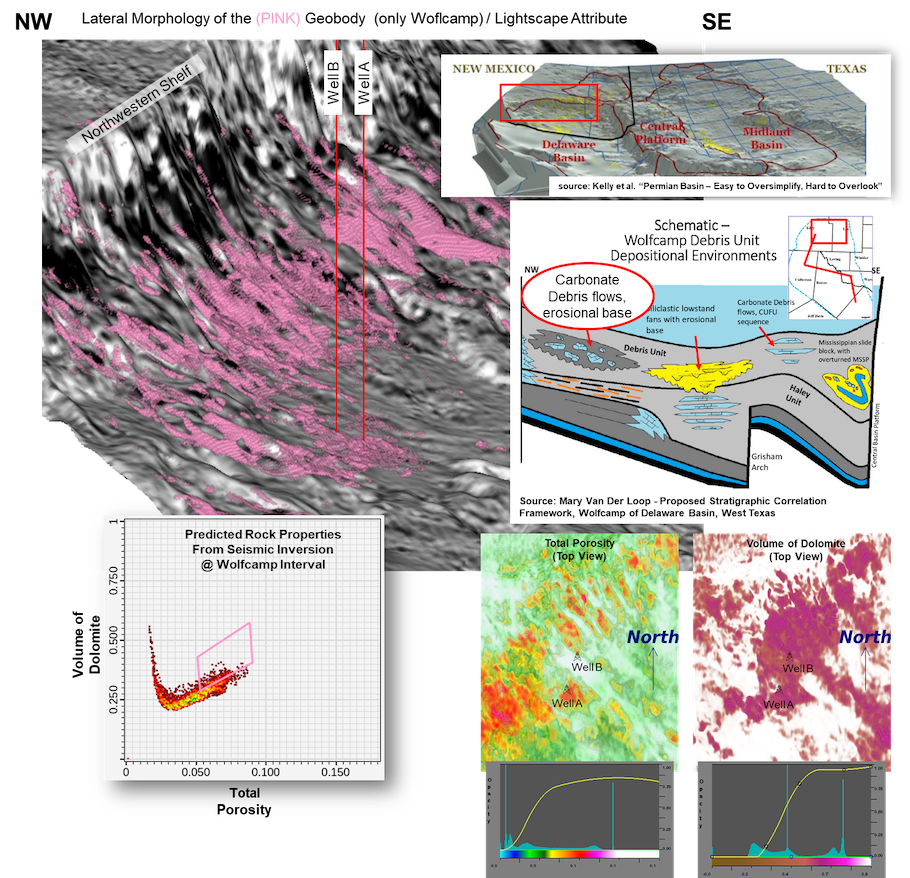
A better understanding of the internal geometry of these complex debris flows was only possible by combining the two rock property volumes because the stacked seismic amplitude data by itself did not provide such amount of details. Additional cubes like volume of calcite, volume of illite, etc. were also predicted and used to support the results shown in this study. The use of these rock properties, and any additional rock/mechanical property predicted through this method, extends to populate static models prior dynamic simulations, uncertainty analysis and reserves estimation, among others.
RELATED CONTENT:
Rock Physics Diagnostics: A Prerequisite for Successful Modeling
Artificial Intelligence Platform Accelerates Reservoir Opportunity Identification
Integrating Seismic Prestack and Well Data Augmented through Machine Learning
Recommended Reading
Halliburton’s Low-key M&A Strategy Remains Unchanged
2024-04-23 - Halliburton CEO Jeff Miller says expected organic growth generates more shareholder value than following consolidation trends, such as chief rival SLB’s plans to buy ChampionX.
Vår Selling Norne Assets to DNO
2024-05-08 - In exchange for Vår’s producing assets in the Norwegian Sea, DNO is paying $51 million and transferring to Vår its 22.6% interest in the Ringhorne East unit in the North Sea.
SLB OneSubsea JV to Kickstart North Sea Development
2024-05-07 - SLB OneSubsea, a joint venture including SLB and Subsea7, have been awarded a contract by OKEA that will develop the Bestla Project offshore Norway.
For Sale, Again: Oily Northern Midland’s HighPeak Energy
2024-03-08 - The E&P is looking to hitch a ride on heated, renewed Permian Basin M&A.
E&P Highlights: Feb. 26, 2024
2024-02-26 - Here’s a roundup of the latest E&P headlines, including interest in some projects changing hands and new contract awards.