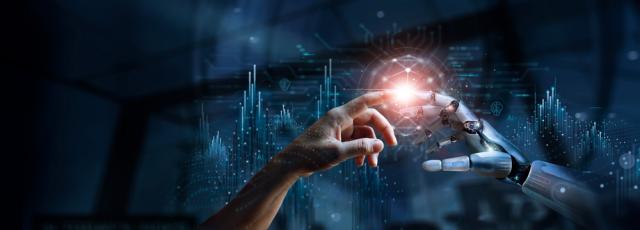
Fueled by massive amounts of data, oil and gas players are using technologies such as machine learning and artificial intelligence to drive efficiency, lower greenhouse-gas emissions and better manage CO2.
For some, artificial intelligence (AI) and machine learning (ML) conjure up fears of humans replaced at job sites.
However, Kayla Ball, chief product officer for Validere, doesn’t see that happening for the oil and gas industry.
“I think we run extremely lean as it is as an industry, and these capabilities are just here to supercharge us,” Ball said of ML and AI. Instead, she sees these technologies playing roles in predictive maintenance, helping to manage carbon based on data collected in the field.
“I think you start to see tiny elements of it right now in terms of…tank pressure readings, choking back the wells so you’re not killing the wells, automating things like that where you used to have to send someone out on site to do it.”
That frees up the time to focus on more tasks that add value and profit for companies, she said during Hart Energy’s recent Carbon & ESG Strategies conference.
Fueled by massive amounts of data, oil and gas players are using technologies such as ML and AI to drive efficiency, lower greenhouse-gas emissions and better manage CO2. The infusion of smart technology comes amid a global push to lower emissions to slow global warming. Companies are putting these technologies to use in many ways—not only to minimize risk and unlock valuable insights, but ultimately deliver returns.
Putting AI, ML to use
Consider the subsurface: Texas A&M University professor Siddharth Misra sees AI as a useful tool for real-time visualization and monitoring of that largely hidden terrain.
Likewise, AI can make sense of high-resolution seismic imaging technics, known as full wave inversion, and apply them to geological carbon storage.
“If you want to monitor anything in the subsurface, you have to use full wave inversion. Full wave inversion with a large high-fidelity simulator is next to impossible. So, for that, AI becomes a tool that accelerates the full wave inversion,” Misra said.
In addition to real-time monitoring, Misra said, AI can be of assistance to engineers and geoscientists for “rapid forecasting when it comes to injection or production optimization, while managing water production.”
AI tools have also shown promise in selecting the right solvents for a particular operating condition for chemical-based carbon capture systems and helping to design “new, novel absorbents which are resistant to water, which are resistant to impurities in the gas from which you want to extract the CO2,” Misra added. “So, this is a pretty powerful use case of AI as a tool for carbon capture, which ultimately helps in carbon management.”
For daily operations, use of the technology to optimize predictive maintenance could also factor into scheduling, particularly when to send people into the field, added Dale Erickson, intelligent operations technology development lead for consultancy and engineering firm Wood Plc.
“It’s both reducing, potentially, the carbon footprint if you’re optimizing their route, but also [it’s] a safety thing… You can reduce driving miles,” he said.
While potential use cases for oil and gas are plentiful, AI holds promise for other parts of the energy sector. Grid reliability came to mind for Erickson. He pointed out grid challenges and how some green energy is being wasted. AI could be used to help optimize power cycles, balancing energy supply and demand, he said.
“That’s a potentially easy win if you’re not wasting energy because power plants have to stay on baseload,” Erickson said.
Data quality
Al and ML technologies are not without risks and challenges, panelists agreed. For starters, quality data is key. And, getting rid of bad data is a must.
“There’s a lot of the Johnny come lately of, ‘hey, let’s slap some machine learning on top of our datasets.’ …The key to all of this is the quality of the data that we have going in,” said Elliott Chorn, executive vice president of product for Amperon, which applies real time operational analytics to power grids. “That’s the key to all of this. None of these large mathematical models work without data.”
The models are trained on data, but datasets must be in one place to make it work, Ball added. Looking at day-to-day interactions across upstream, midstream and downstream, “it’s not an AI problem yet,” she said. “The problem at the end of the day is that all of the data, go figure, are still in silos. EHS [environmental health and safety] and compliance teams are working off a particular portion of the data.
“Operations teams are looking at other portions of the data; finance, corporate sustainability, and the amount of data that’s being collected is significant in the field. No one is really aggregating it into one place.”
Until that happens, correlating data from flare stack thermocouples and flow meters with readings from optical gas imaging cameras, for example, will be a struggle when trying to build a predictive model, she said.
Looking ahead
Another big risk, Misra said, is that models may give you an “amazing result on your training data, but they may not ever generalize in the real world” because they tend to overfit as they go into deeper neural networks. This means predictions become unreliable in a real-world scenario, Misra said.
“Edge cases are where things really start to fall apart. We’ve been seeing a lot of that,” Chorn said, turning to high energy demand in the Electric Reliability Council of Texas, or ERCOT. “As we start to see some of these extreme weather patterns, we’re starting to get out on the edge. We don’t have good data to correlate back to and real challenges emit from there.”
Despite such complexities, panelists see more future uses for AI and ML in energy. These include mapping subsurface changes to avoid induced seismicity and automation of more manual tasks prone to human error.
“The future is really what I’m excited about because it’s been kind of an edge science that was happening, again, behind the scenes, but not that forward facing,” Chorn said. “Now, it’s become forward facing; it’s become sexy, it’s become cool, and that’s where real innovation and exciting things are going to come from, in my opinion.”
Recommended Reading
Exxon Mobil, Chevron See Profits Fall in 1Q Earnings
2024-04-26 - Chevron and Exxon Mobil are feeling the pinch of weak energy prices, particularly natural gas, and fuels margins that have cooled in the last year.
Oil and Gas Chain Reaction: E&P M&A Begets OFS Consolidation
2024-04-26 - Record-breaking E&P consolidation is rippling into oilfield services, with much more M&A on the way.
Matador Stock Offering to Pay for New Permian A&D—Analyst
2024-03-26 - Matador Resources is offering more than 5 million shares of stock for proceeds of $347 million to pay for newly disclosed transactions in Texas and New Mexico.
Aramco Reports Second Highest Net Income for 2023
2024-03-15 - The year-on-year decline was due to lower crude oil prices and volumes sold and lower refining and chemicals margins.
Weatherford M&A Efforts Focused on Integration, Not Scale
2024-04-25 - Services company Weatherford International executives are focused on making deals that, regardless of size or scale, can be integrated into the business, President and CEO Girish Saligram said.