The data landscape is messy.
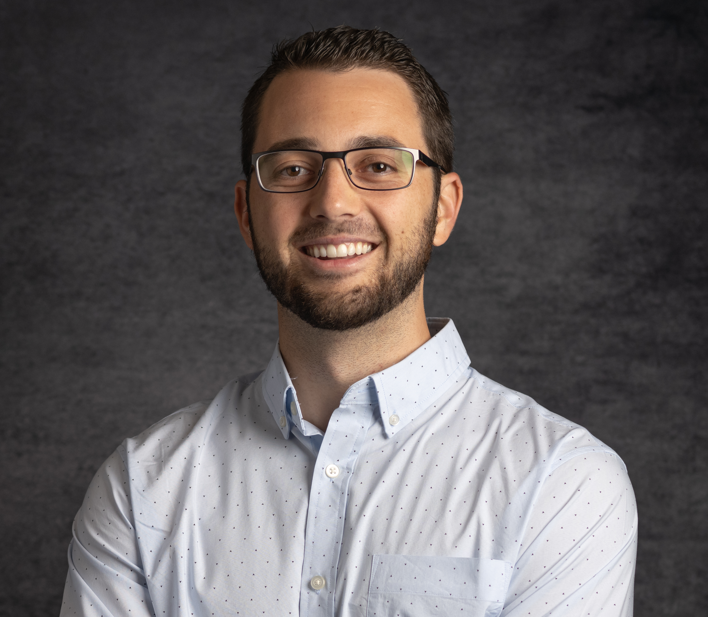
Data lives in silos—in apps, on paper, in enterprise resource planning software and engineering drawings and documents, and maybe even a few desk drawers.
These scattered sources make it difficult to locate needed information quickly and get it to the right people and applications to make the best use of the data, Cognite’s Ben Skal, senior director of product marketing, told Hart Energy.
He said the “data landscape mess” is partially due to the proliferation of applications that solve individual problems, such as predictive maintenance, but add a layer of complexity in some other way. The convoluted ways in which data is stored makes it “really, really difficult to scale,” he said.
“A new approach is needed,” he said.
According to executives responding to Cognite’s “Asset-Heavy Industry Survey: Simple Access to Complex Industrial Data” report released on Sept. 27, it is difficult to trust industrial data. Only 3% of respondents said they completely trusted their critical decision-making data, while 50% said they were at a three on a scale of one to five in trusting data.
With 97% of the respondents saying there is some level of mistrust in data, it’s worth thinking about what the 3% are getting right, he noted.
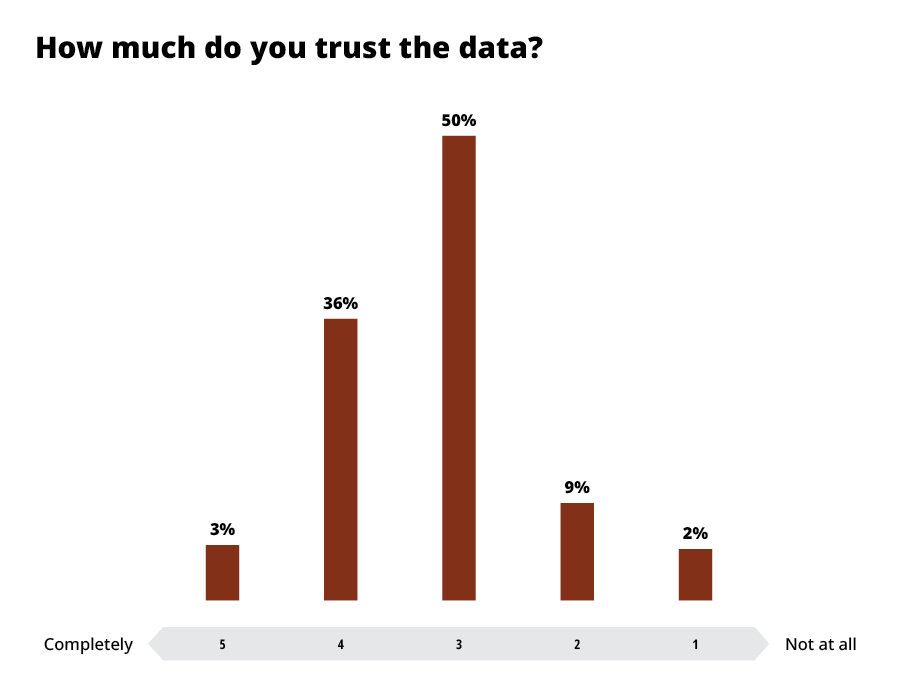
“It's one thing to be able to bring it together, but it's another thing to be able to understand how time-series data is related to equipment, is related to documents, is related to the drawings” representing operations, Skal said. “I would say what that 3% is doing is recognizing that it's not just about connecting, it's about understanding and providing that data in an interactive, user-friendly way to their end users who actually make decisions.”
According to the survey, 56% of executives cited unaligned data scattered across their systems as a key challenge. Another 50% said they had no holistic view of their data, which made it difficult to gain insights or collaborate across teams. About a third cited implementing data management systems at scale, the amount of time it takes to get the right data to the right people, and discrepancies between reported data and actual situations as challenges to managing data.
Currently, top priorities for executives are to improve production.
With those challenges in mind, around half of surveyed executives are prioritizing improving production output, reducing maintenance costs and reducing the risk of critical asset failures.
They’ll need data to do those things, and Cognite sees unifying industrial data across the operation technology (OT), information technology (IT) and engineering landscapes as the way to deliver on those goals, Skal said. And generative artificial intelligence (AI) will play a role, he added.
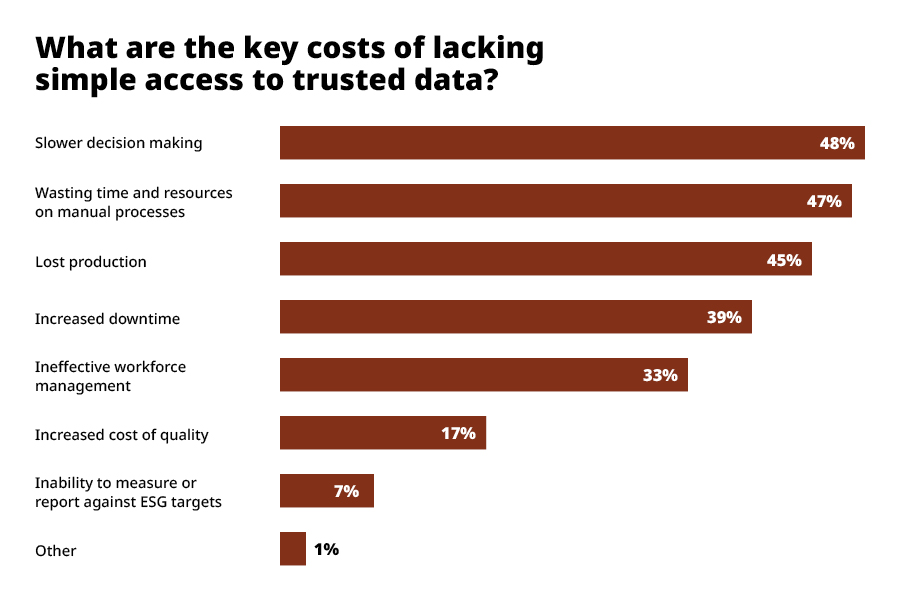
In the field, putting together the pieces of the puzzle requires bringing context to data when a user accesses it. For example, a worker in the field spotting an anomaly with a piece of equipment can use a mobile phone to take a picture of that equipment’s tag. A search engine powered by generative AI can return relevant information about the equipment, such as the maintenance history, the original equipment manufacturer’s documentation on the equipment, troubleshooting recommendations and other data.
To combat the issue of generative AI’s tendency to “hallucinate” or deliver inaccurate information, Skal noted, responses include source materials to enable verification of information.
“We're not expecting people to trust generative AI results on their own,” he said. “It's about creating that transparency for where those results came from.”
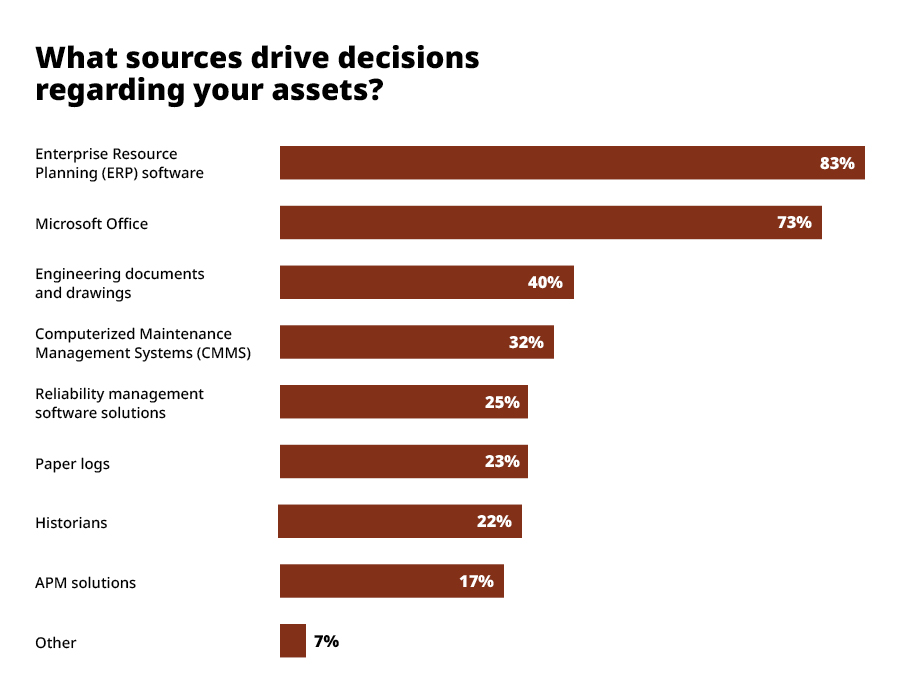
A search service powered by generative AI in a connected worker app called InField is currently in beta test with an upstream oil and gas company and is expected to become widely available to the industry by the end of the year, Skal said.
Companies interested in progressing their digital journey should be linking data problems with business problems, he said. It can be tough to get buy-in to fund a data clean-up project when the company needs to improve production, he said. But approaching the business problem in a thoughtful way can contribute to cleaning up the data, he said.
“When you think about it like a step change in a journey, then all of a sudden, this idea of ‘how do I start solving that industrial data problem’ becomes a lot more manageable,” Skal said.
Recommended Reading
Occidental Increases Annual Dividend by 22%
2024-02-11 - Occidental Petroleum Corp.’s newly declared dividend is at an annual rate of $0.88 per share, compared to the previous annual rate of $0.72 per share.
Atmos Energy Announces Quarterly Dividend
2024-02-11 - Atmos’ dividend marks the company’s 161st consecutive quarterly dividend.
From Restructuring to Reinvention, Weatherford Upbeat on Upcycle
2024-02-11 - Weatherford CEO Girish Saligram charts course for growth as the company looks to enter the third year of what appears to be a long upcycle.
Atlas Energy Solutions Declares Dividend
2024-02-09 - Atlas Energy’s dividend represents a 5% increase from the previous quarter’s dividend.
Northern Oil and Gas Ups Dividend 18%, Updates Hedging
2024-02-09 - Northern Oil and Gas, which recently closed acquisitions in the Utica Shale and Delaware Basin, announced a $0.40 per share dividend.